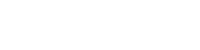
Binary Minds | A.I. in Healthcare
Episode 2 | 26m 46sVideo has Closed Captions
Explore how A.I. is being incorporated into healthcare and what it means for the future of medicine.
Artificial Intelligence is being incorporated into healthcare from chatbots to cardiac care. Explore what the future of medicine with A.I. holds with medical professionals, ethicists, and computer engineers.
Binary Minds is a local public television program presented by WKAR
Support for Binary Minds is provided by MSU Research Foundation
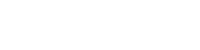
Binary Minds | A.I. in Healthcare
Episode 2 | 26m 46sVideo has Closed Captions
Artificial Intelligence is being incorporated into healthcare from chatbots to cardiac care. Explore what the future of medicine with A.I. holds with medical professionals, ethicists, and computer engineers.
How to Watch Binary Minds
Binary Minds is available to stream on pbs.org and the free PBS App, available on iPhone, Apple TV, Android TV, Android smartphones, Amazon Fire TV, Amazon Fire Tablet, Roku, Samsung Smart TV, and Vizio.
Providing Support for PBS.org
Learn Moreabout PBS online sponsorshipWkar is supported by the MSU Research Foundation, bringing new innovations to the marketplace for global impact.
Learn more at MSUfoundation.org The health literacy and health care with AI is a very complicated issue.
We don't really have enough health literacy to comprehend our own health conditions.
How do you get large amounts of data and translate that into something that can benefit individual patients?
And that's something that the power of AI can really help with.
How can we use artificial intelligence so we can treat that patient even faster than before?
People are using generative AI tools to take tasks that used to take them hours to do, and they're compressing it into minutes to do.
Artificial intelligence offers, in part, enormous promise for medicine.
But at the same time, there are a number of ethical issues that are raised by the use of AI in medicine.
There are multiple definitions of artificial intelligence.
Suffice to say that in today's world, artificial intelligence is kind of a catchall phrase.
Artificial intelligence describes a range of technologies that imitate any form of human intelligence.
AI is structured in a way to mimic what are called the neural networks that define our own brain.
Just like neurons have a set of inputs, and then they selectively activate or fire in a similar way.
We have these artificial versions of those neurons that we string together to become sensitive to certain kinds of images, or certain things they observe in a signal, or they observe in text to activate so that they can write poetry, tell stories, create images, and so on.
At the core of developing these AI methods is obviously data.
Giant amounts of data.
Data has to inform the AI.
It has to inform the algorithm.
And that's how the algorithm learns in order to produce a particular output.
An answer to a question.
And it can also do that by learning on its own how to identify patterns, and then act on the basis of those patterns.
There are three main advantages to artificial intelligence.
The first is AI can provide valuable insights by taking complex systems and distilling information out of them.
Healthcare has a lot of information A lot of data points genomic meaning your DNA data.
You have, your past history, your family's history, and there's a lot for a provider to have to come together to really understand the person and then to navigate what kind of care they need.
A few years ago, we started exploring the option of how to integrate AI into health care, and we selected coronary artery disease its the number one killer in the US.
If we can intervene on the disease early, we have a chance of not only preventing heart attacks and strokes, but also potentially reversing the disease.
What we were able to find then was a solution where the AI data was integrated into our electronic health record.
That health record then would search the patient's database to see if there had been a prior diagnosis of coronary disease for those patients that had high coronary calcium scores.
Then the algorithm would send a notification to the patient's primary care physician.
The algorithm would also give recommendations as to treatments, further testing, and possibly the need for a referral to a cardiologist if necessary.
AI can also be used to augment people making work less tedious.
What AI is allowing us to do is to take some of those menial tasks that we do day in and day out, and we can shift those to AI to allow then our team members to focus at the top of what they do.
So for provider, for example, they might spend 5 to 6 hours a day documenting those visits with patients.
If we can use AI to capture the conversation between patients and providers.
Create a note that the provider can utilize, edit, and then accept that's less time that they have to spend on documenting and more time on the care delivery of the patients.
My laboratory at Michigan State works on methods that combine human and machine intelligence to accomplish what neither humans nor machines can do as effectively alone, so we're very interested in that augmentation problem.
One example of this is if you're a physician who is in a hospital setting, and you have to dose a set of very sensitive medications, sedatives, anticoagulants, and so on.
What we'd like to do is have the machine figure out, based on the characteristics of the patient, how we can recommend the optimal dose so that you don't oversedate.
You don't undersedate still make the final dosing decision on these medications.
But we want to equip them with the knowledge and the tools and the insights that the AI systems can provide so that they can deliver the care most effectively.
Among the more promising aspects of AI in medicine is the ability of artificial intelligence to read medical images.
AI is able to aggregate and look at information on images, X-ray, radiology, mammography tests to really bring out suspicious activity that maybe a provider might not be able to see.
In some studies, 60% of heart disease was missed by the human being reading the Cat scan.
AI being able to do pixel level data can really wring out information from those scans that we wouldn't be able to do just with human visualization.
A case that's been written up is the case of Barbara.
We can only use a first name here.
She was being checked for breast cancer, and her MRI scan was read by two radiologists who really didn't see anything problematic there.
But they decided that they would put it through AI.
And sure enough, AI identified a stage two breast cancer that could have had a fatal outcome for her if, in fact, that cancer had been allowed to develop without treatment.
So there's been some research study that has been done to find out.
Does AI improve the diagnostic quality that radiologists are capable of?
And the research actually gave us kind of a mixed picture.
One of the things that showed was that if a particular radiologist is not as good as most other radiologists, then AI is not going to improve by very much the low skill level of that particular radiologist Its at least as important to have very well trained radiologists using AI to get the kind of positive therapeutic result, diagnostic results that we would expect.
There are also many issues and concerns surrounding the deployment of AI in healthcare.
One of the biggest downsides that I think most of us in the field do recognize is its accuracy.
AI, at the end of the day, is not another human brain.
It's an algorithm created by humans based on human data.
The quality of the data, the integrity of the data is what will determine the quality and integrity of what the output is from AI.
There's a risk with AI that if you teach it, inappropriate results, inappropriate information, fake information, it can, in a confident manner give you an output that you might believe in.
We designed these AI to produce the most average answer, right?
It doesn't produce the truth.
It doesn't know what the truth is.
It's a machine, right?
So you need to have a human being overseeing the AI algorithms output to verify that the AIs interpretation of the data was accurate.
And that becomes an ongoing process.
The substance of the data being fed into these AI systems isn't the only point of contention.
Where and how the data is sourced are also concerns.
AI is based on data sets.
Those data sets are extracted from our world.
And of course we live in and have historically lived in a pretty unequal and unjust world.
And therefore the data that AI is based on is unequal and unjust, and as a result, it's producing output that reinforces those biases.
And it does so often without us even realizing it, because it seems like it's an objective technology.
So how could it possibly be biased?
The issue of bias in AI algorithms is something that the research community is aware of, and I think a lot of people are taking this very seriously.
We want to solve it.
the way that the data gets generated or curated to go into these models depends on who's building them.
The people who build the AI play a very large role in determining what kinds of data the AI should pay attention to.
In the case of human medicine, every health care system that is deploying AI is going to have to verify that the algorithm based results are indeed accurate for the patient population that they're serving.
If I train my AI on a population of 24 year old white males, and I want that AI to enact itself across a very good, diverse and equitable demographic ecosystem, that tool is going to have a bias in it and drive you down a path that is inappropriate.
So understanding those training models then gets you to that point of where is it being used?
How are you ethically monitoring it, watching it, and really making sure that humans in the loop understand where there might be risk and where they might have to understand and train the AI model to be more effective in its use.
One question would be to what extent do the users of the algorithm, the people who are interpreting that data, to what extent did they have the opportunity to weigh that against their knowledge in areas where there are, you know, sort of long standing questions about inequity and injustice.
You might ask, To what extent are users of these algorithms trained in implicit biases and other kinds of, you know, sort of histories of structural inequality so that they know about the potential problems of the algorithm, but also the potential problems with their own interpretations of those algorithms.
People don't want to build systems that are discriminatory or biased or hurt people.
And there's been some very serious efforts to design the next generation of AI systems.
So it's aware of what matters and what doesn't so that they can avoid that bias.
That's the area that there's a lot of activity in the research community on, really exciting activity that I think will continue to solve this important problem.
People looking for health information online are particularly vulnerable to some of these issues of algorithmic bias.
Each and every day Google is used for medical purposes.
I have to believe that the vast majority of those uses are by all kinds of Americans who don't realize that Google itself represents a form of AI technology.
They are more likely to see information that is personalized to their habits and their past history, and not necessarily see information that's credible medical advice.
And the basic problem with that is that if individuals think that they have confidently figured out their own medical problem, they will fail to actually see a physician who is capable of appropriately assessing the information.
And that can have disastrous consequences for patients.
Understanding the inner workings of AI models is crucial for transparency and accountability, leading to questions about the path the AI systems take to produce their output.
In my field, we talk about all technologies as being what we call black boxed.
And when we talk about the language of black boxes, we say that any technology once it goes out into the world, the users as well as the developers tend to treat it as a black box, as though we don't totally know.
We don't need to know.
But something great happens and it, you know, performs a function that we can't do on our own.
The most classical version of these artificial intelligence systems, straightforward machine learning approaches, didn't really have this problem.
We could peel back the onion all the way to the core, totally understand exactly each of the steps in the decision making process that the AI was using.
What's transitioned more recently is we've given more autonomy to the machines to learn what matters when it's generating its responses to emulate actually what human beings do.
If I asked a person to to provide a justification or a reason for why they made a decision, a justification can be produced.
Now, the extent to which that justification encompasses the the absolute underlying reason is an open question.
The reality is that it's really important to open up that black box and understand what are all of the assumptions and values and biases that are embedded in those seemingly objective processes.
And to make sure that it's a technology that's really serving us well and not doing harm.
We usually take it to be the case that the first ethics commitment of a physician is to do no harm, but in order for that to be the case, the physician has to know what do I need to do to avoid harming my patient?
And among other things, the physician must know confidently why this diagnostic judgment is most likely a correct judgment, or why this therapeutic recommendation is most likely a sound kind of therapeutic recommendation.
The problem with the black box and the use of AI is that the physician really doesn't know exactly how AI came to this particular recommendation, or this particular diagnostic judgment.
I think that leads into the conversation about AI should never stand on its own.
You should always have a human in the loop that is a subject matter expert on what that AI is delivering.
So then they can really have an understanding of why it was used in a certain manner.
Before we started deploying AI into our clinical space.
We did have concerns about what the patient acceptance of AI would be, and so we did patient focus groups before we started.
Patients in the exam room are able to see what the AI did.
We can show them their own images and help them understand what that means for them.
As possible threats from artificial intelligence grow.
The world is racing to construct balanced regulation around AI that protects citizens.
One of the biggest fears that we have, as as I guess, a society when we talk about artificial intelligence is can it get ahead of us?
Is it going to get ahead of us?
The technology is actually moving faster than what we're able to do as far as keeping up with governance, keeping up with policy and procedure.
The way that it's operating now, the way that the industry is developing now, it's developing, let's be honest, according to market values, that is what the consumers want and what the producers want.
This is maybe the first time in history, as far as I can see, where you have this cutting edge technology, but it's been completely spearheaded by private corporations, unlike wireless or the internet, which was all because of governmental initiatives.
It's a question of public welfare and who is getting the benefits from these technologies?
Are they going to serve the interests of the greater common good?
There will definitely be some sort of action in the future to regulate AI systems.
A lot of the federal agencies, not just in the US, but also internationally, are already taking steps to put the guardrails or boundary conditions on how these systems can be used and for what purposes.
In addition to regulation at the national level, we need to think about whether or not we should engage in regulation at the state and local level.
We're seeing that on a piecemeal basis.
You've had Illinois that's passed biometric privacy regulations.
You have some cities that have also done sort of little bits of regulation around what can and can't be allowed.
But the truth is, is that a lot of states and, and localities don't have the capacity or the funding to actually vet technologies in the way that they really need to.
And that's why national governments have a really important role to play.
I do feel that there needs to be oversight and some regulation within artificial intelligence, but I think there's also a good conversation around that also needs to have its checks and balances.
There's really a fine line about regulation and hurting innovation, and helping us be more comfortable and more trustworthy in the innovation.
In our founding document, the Constitution, we famously talk about the importance of intellectual property, really as a means to stimulate economic growth in the country.
Maybe 200 years ago, technology was a little exotic for us.
But now technology is part of all of our lives, and so we're interacting with it all the time.
We're very aware of the limitations of technology.
This is a moment, I think, where we see that innovation is about way more than just economic growth.
And that's why it's so important to consider that and bring it into regulation, because I think that if governments don't do that, then there are real risks of losing public trust, not just in technology, but also in government too.
The use of artificial intelligence in healthcare comes with a number of financial implications.
Let me give you one example.
Right now, MRI scans can be used to identify the earliest stages of cancer by doing whole body MRIs.
The cost of that is $1,300.
One company is saying, we want to get the cost of doing a whole body MRI down to $500, and then we want everyone in the United States over the age of 40, to have the opportunity to have one of these scans on an annual basis, looking for cancers.
That would be 200 million Americans at $500 apiece each year, that represent $100 billion in costs, and for 99.5% of those individuals every year, they would be told, “you don't have cancer” but we would have spent $100 billion to find that out.
So we have to ask ourselves, as a society, is that a good use of limited healthcare resources?
Is that something that insurers would want to endorse?
And as things are right now, insurers are reluctant to actually pay for the use of this technology because from their point of view there is too much uncertainty, there's too much lack of transparency.
The black box issue, again, for them to say that there's going to be a good outcome here from their point of view, that is, it's going to save us money.
There's a case, for example, of a health insurer who used an algorithm to basically shape how many days it would pay for extended care.
This was for elderly patients and for disabled patients.
And they basically said the algorithm says, I mean, they didn't tell the people.
They had to kind of ferret out that the algorithm was being used, but they were basically using this algorithm to restrict the amount of payment that the insurance company would make.
And it wasn't just a problem with the algorithm allowing only a limited number of days for reimbursement.
It was also that the employees of the company were not allowed to overrule the algorithm, right?
So they were incentivized to basically follow what the algorithm said.
I do think that we could be on the precipice of where these tools are used in a conflict sort of manner within our ecosystem, where an insurance company says, well, AI says this, and our providers say, well, time out.
This is our demographic.
I think this is where we want to educate them to talk about, okay, let's understand the use of your AI tool insurance company.
What model is it built on?
What tool sets is it built on?
What training has it had?
What demographic is it using?
And having those conversations.
The funny thing about that is in health care, we're used to that, asking those questions, because there's a lot of outside entities telling us how to deliver care, because there's a lot of players in the in the health care world.
And I think that that's where we have to continuously focus on training, continuously focus on transparency and dialog when those tools are being used and asking probing questions.
Where was it used?
How was it used?
Why was it used?
As artificial intelligence becomes more integrated into daily life It's essential to determine the role AI will play in shaping society.
I think that part of the crisis that we have in our country right now is because a lot of us feel powerless and we feel like we there are problems that are beyond our control.
But at the end of the day, the solution is to work together, to have conversations about it and to realize that we all have power to make a change, and that the people who can make those changes aren't just the technologists, and they aren't just the government.
So if we as citizens feel empowered, then they'll be forced to figure out the answers.
And that's where we start I think.
We actually have to think through some of these challenging ethical questions, and it's going to take a lot of partnership between people who build the core technologies.
Folks like myself and computer sciences, but also people who think deeply about about ethics and also for the broader society.
These are questions not just to be answered within the hallowed halls of a university or within Congress, but they need to be discussed with the public.
The public has to weigh in on these things as well, and figure out what are the right ways to behave.
The health care system is being very focused and very intentional about artificial intelligence, having patients at the table about how you're implementing it.
Having an advisory council to talk through these avenues of how it's being used.
I would encourage patients to ask their physicians if AI is involved in their care, and if so, at what level.
The more patients know about it and how it's being deployed, the more comfortable they're going to be with it.
So what does the future hold for artificial intelligence in medicine?
It's such a hard answer because it's being used today in something I didn't think of yesterday.
I think you're going to start seeing more of the artificial intelligence being embedded more in our physical presence.
You're going to start seeing artificial intelligence sensors, radar, heat detection, to monitor that room so we can treat that patient even faster than before.
We could have an android that helps care for elderly people who need to be lifted out of bed in the morning.
An android that helps the ill.
There's all these really wonderful, very positive things that we can do with this technology.
Now, that also comes with risks like any exciting technology does.
If we keep going the way we're going, I fear that we're going to incorporate AI into our lives in a pretty thoughtless way.
And I think that it's really important to bring that human involvement back.
That's one place where we can make sure that the harms are limited and the benefits are amplified.
One of the questions that patients ask us is, where is AI going to fit in the future in health care?
Where we really see AI working is in the background, assisting the physician to help improve their ability to manage their patient's care.
And our hope is that we'll be able to involve our patients in that development process, that it's something that we're going to explore together with our patients.
At the end of the day, the physician patient relationship remains paramount.
WKAR is supported by the MSU Research Foundation, bringing new innovations to the marketplace for global impact.
Learn more at MSUfoundation.org.
{Jaunty Piano Jingle}
Video has Closed Captions
10pm ET Thu NOV 14, 2024 on WKAR TV | The implications of artificial intelligence on Healthcare. (1m)
Providing Support for PBS.org
Learn Moreabout PBS online sponsorshipBinary Minds is a local public television program presented by WKAR
Support for Binary Minds is provided by MSU Research Foundation